Limited Resources: Budget Constraints Affecting Access to AI Tools, Training, and Hiring Specialized Staff
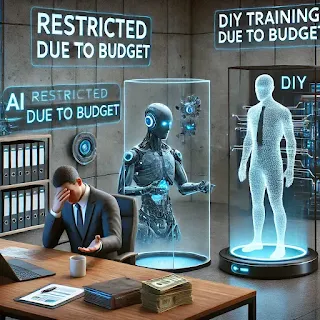
Introduction: The Critical Role of Resources in AI Adoption
Artificial intelligence (AI) is not just a buzzword but a transformative force reshaping various domains, including libraries. The potential of AI to revolutionize information retrieval, enhance user experiences, and streamline operational processes is attractive and exciting. This transformative force of AI is what we are all eagerly anticipating. However, the integration of AI technologies is challenging, particularly when it comes to resource allocation. Libraries, especially those operating within budget-constrained environments, face significant hurdles that limit their ability to access AI tools, invest in training programs, and recruit specialized staff. This analysis explores the multifaceted impact of resource limitations on AI adoption in libraries. It offers insight into how these barriers manifest, perpetuate, and potentially exacerbate inequalities in access to cutting-edge technologies.
Budget Constraints Limit Access to AI Tools
The implementation of AI in libraries often starts with the acquisition of appropriate tools and software. However, the high costs associated with these technologies present a significant obstacle. AI tools, from natural language processing (NLP) algorithms for cataloging to machine learning models for predictive analytics, demand substantial investment. Recent studies in library sciences reveal that the average cost of deploying AI systems can range from tens to hundreds of thousands of dollars, depending on the scale and sophistication of the implementation (Smith & Lee, 2023). This financial burden is significant for many libraries.
High Initial Costs
The initial financial outlay for AI technologies includes hardware, software licenses, and infrastructure upgrades. For example, high-performance servers and cloud-based computing systems are often prerequisites for running AI algorithms effectively. Many libraries, particularly public and community institutions, operate on shoestring budgets that prioritize essential services over technological experimentation. As a result, AI adoption remains an aspirational goal rather than a tangible reality for many institutions.
Ongoing Maintenance Expenses
AI technologies demand consistent maintenance to ensure optimal performance. This includes software updates, hardware replacements, and cybersecurity measures. These recurring costs can further strain library budgets, making it challenging to justify AI investments compared to other pressing needs, such as book acquisitions, staffing, or building maintenance. The long-term financial commitment required for AI technologies is a significant consideration for libraries.
The Digital Divide
The disparity in resource allocation among libraries exacerbates the digital divide, with well-funded institutions benefiting from AI advancements while underfunded libraries lag. This phenomenon creates a two-tiered system where access to AI-driven services becomes a privilege rather than a universal standard. The digital divide directly impacts the quality and equity of library services.
Insufficient Funds for Training Programs
No matter how advanced, AI tools are only as effective as the individuals using them. This underscores the urgency and importance of training library staff to harness the full potential of AI technologies for successful implementation. However, the cost of professional development programs often proves prohibitive for resource-constrained libraries.
High Cost of Professional Development
External organizations or tech companies typically offer workshops, certifications, and specialized training programs in AI, with fees ranging from hundreds to thousands of dollars per participant. These expenses often exceed the training budgets of many libraries, forcing institutions to either forego training altogether or rely on limited, in-house resources.
Impact on Staff Morale and Retention
The lack of training opportunities can lead to frustration among staff who feel ill-equipped to handle new technologies. This hampers the effectiveness of AI adoption and contributes to job dissatisfaction and higher turnover rates. In contrast, well-funded libraries that invest in training create environments where staff feel empowered and engaged, further widening the gap between resource-rich and resource-poor institutions.
Long-Term Implications for Institutional Knowledge
With adequate training, libraries can avoid developing a superficial understanding of AI capabilities, which can lead to underutilization or misuse of tools. This can result in a vicious cycle where poorly implemented AI projects fail to deliver value, reinforcing skepticism about their efficacy and discouraging future investments.
Challenges in Hiring Specialized AI Staff
Successful deployment and management of AI systems require specialized expertise. However, hiring data scientists, machine learning engineers, or AI specialists is often beyond the financial reach of most libraries, particularly those in rural or underserved areas.
Competitive Salaries in the AI Sector
The demand for AI professionals far exceeds the supply, driving up salaries in the tech industry. Libraries, which traditionally operate on limited budgets, need help to compete with private sector organizations offering lucrative compensation packages. This creates a talent gap where libraries must help or retain the necessary expertise for AI initiatives.
Reliance on Multitasking Staff
Without dedicated AI specialists, libraries often rely on existing staff to manage AI projects in addition to their regular duties. This approach not only dilutes the focus on AI but also increases the workload on staff, leading to burnout and reduced productivity. Furthermore, the lack of specialized knowledge can result in suboptimal implementation, undermining the potential benefits of AI.
Impact on Innovation and Sustainability
The inability to hire specialized staff limits a library's capacity for innovation. Without the expertise to design, implement, and evaluate AI projects, libraries are less likely to experiment with cutting-edge technologies or adopt creative solutions to complex challenges. This not only stifles progress but also jeopardizes the long-term sustainability of AI initiatives.
Broader Implications of Resource Constraints
The challenges outlined above are not isolated but are part of a broader ecosystem of systemic inequities and institutional priorities. Understanding these broader implications is essential for developing effective strategies to address resource constraints.
Equity and Access
The resource disparity among libraries reflects and reinforces broader societal inequities. Communities served by underfunded libraries could often benefit the most from AI-driven services, such as enhanced information access, personalized learning tools, or improved cataloging systems. We risk perpetuating cycles of exclusion and marginalization by failing to address resource constraints.
Innovation Gap
Resource limitations hinder individual libraries and slow the pace of innovation across the sector. Collaborative efforts to develop open-source AI tools or share best practices will likely fail when many libraries need more resources to participate meaningfully.
Misalignment with Institutional Goals
Libraries are increasingly expected to align their services with the strategic priorities of their parent institutions, such as universities or municipalities. Resource constraints can prevent libraries from meeting these expectations, undermining their perceived value and jeopardizing their long-term viability.
Addressing Resource Constraints: A Path Forward
While the challenges of resource limitations are daunting, they are not insurmountable. By adopting a proactive and collaborative approach, libraries can mitigate the impact of these barriers and pave the way for successful AI integration. Addressing these resource constraints must be urgent, and immediate action is needed.
Advocacy for Increased Funding
Librarians must become vocal advocates for increased funding, highlighting AI's transformative potential in enhancing library services. Building partnerships with stakeholders, including government agencies, philanthropic organizations, and private sector sponsors, can help secure the necessary resources.
Leveraging Open-Source Tools
Open-source AI tools offer a cost-effective alternative to proprietary solutions. Institutions can collaborate with other libraries and tech communities to share resources, expertise, and best practices to overcome financial constraints.
Incremental Implementation
Rather than attempting large-scale AI projects, libraries can begin with minor, low-cost initiatives demonstrating tangible benefits. These pilot projects can serve as proof of concept, building momentum and supporting more ambitious endeavors.
No comments:
Post a Comment
Note: Only a member of this blog may post a comment.